Human-AI Systems /
What Constitutes a Harmonious Human-AI Interaction?
The prospect of constructive collaboration between humans and artificial intelligence represents an exciting frontier in human-computer interaction. Pioneering researcher Cliff Nass illuminated the interpersonal dynamic with technology, showing computers as social actors rather than neutral tools. His influential work highlighted the multilayered relationship between automation and human oversight. Empowered by rapid advances in machine learning, today's AI integrations now provide dynamic assistance rather than fixed input. However, effective integration depends on maintaining balance between user autonomy and machine influence. In 2017, scholar Saleema Amershi coined the term "intelligence assistance" to describe AI as enhancing rather than replacing human judgment, focusing on transparency and mutual understanding. Successful human-AI collaboration relies on thoughtful interface design that builds user trust in complex systems. As automated partners gain more responsibility, researchers like Joanna Bryson emphasize that explanation becomes essential. AI pioneer Andrew Ng advocates developing AI to augment people via "superpowers" rather than replacement, ensuring human values stay central. This emerging era poses exciting challenges for HCI experts – we must progress beyond building functionality alone to crafting empowering user experiences. By balancing controls and customization with smart recommendations, the human-computer relationship can evolve into interacting with assistive partners. When cultivated in harmony, a symbiotic intelligence emerges where the strengths of both humans and machines can shine.
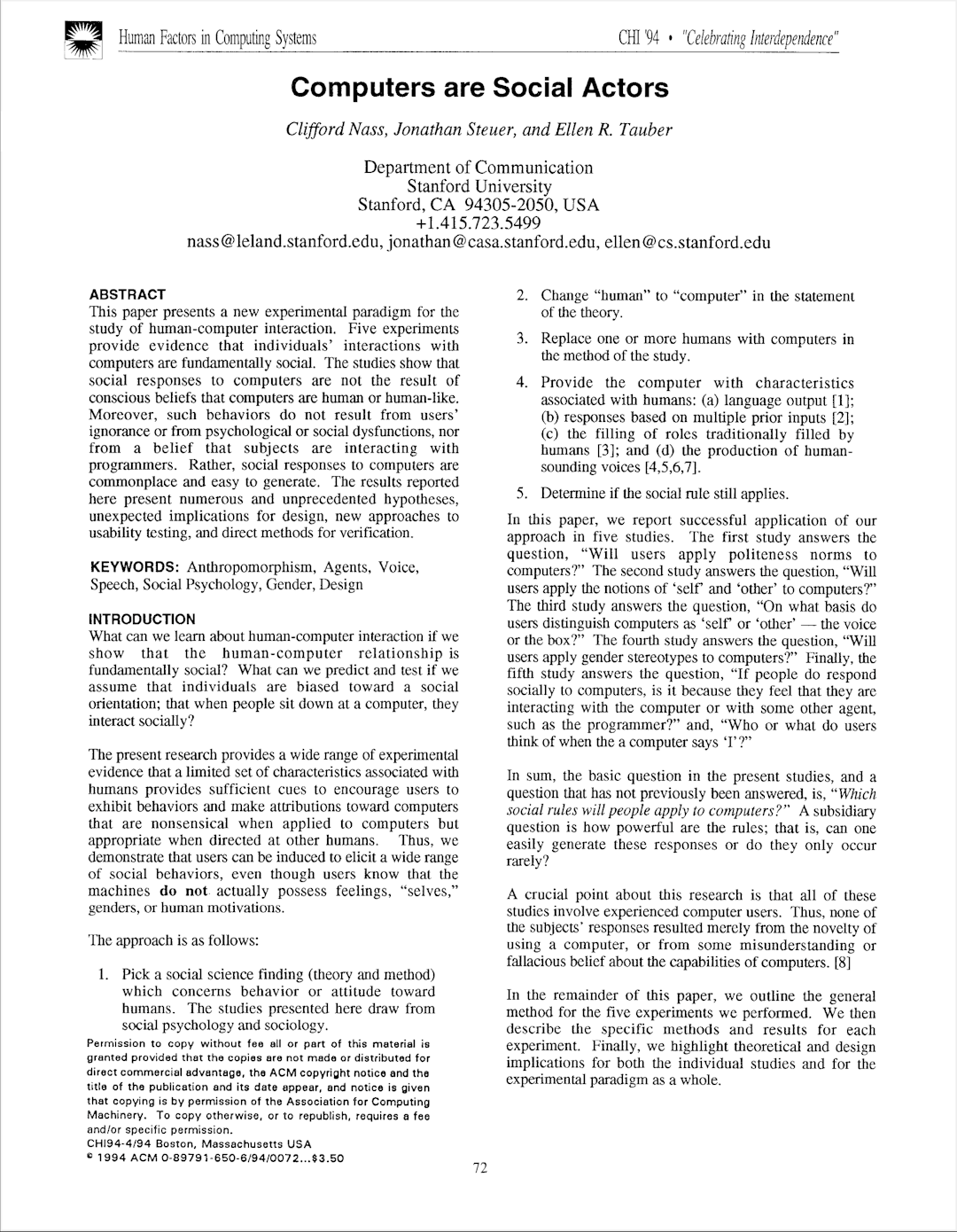
Computers are Social Actors
Clifford Nass, Jonathan Steuer, Ellen R. Tauber · 01/04/1994
This 1994 paper by Nass, Steuer, and Tauber stands as a watershed moment in human-computer interaction (HCI), pushing the boundary of how we perceive interactions with computers. The authors assert that users unconsciously treat computers as social actors, thereby introducing the idea that human-social rules might apply to human-computer interaction.
- Anthropomorphism in HCI: The paper presents experiments showing that people apply social rules and norms, like politeness, even when interacting with computers. This challenges the notion that human-computer interaction is fundamentally different from human-human interaction.
- Social Dynamics: Beyond simple tasks, users attribute personality and even gender to machines. This insight can be used to inform more engaging and intuitive interface designs, making them relatable to users.
- Para-Social Interactions: The concept here is that interactions with computers can evoke emotional responses, similar to interactions with humans. This complicates the interface design process, but also opens doors for more immersive experiences.
- Ethical Implications: If computers are treated as social actors, then ethical considerations, such as honesty and accountability, come into play, particularly in AI-driven interfaces.
Impact and Limitations: This paper significantly influenced subsequent HCI and AI research, driving more sophisticated, socially-aware systems. However, the risks of anthropomorphizing machines—such as overtrust or emotional dependence—remain an area for further study and ethical deliberation.
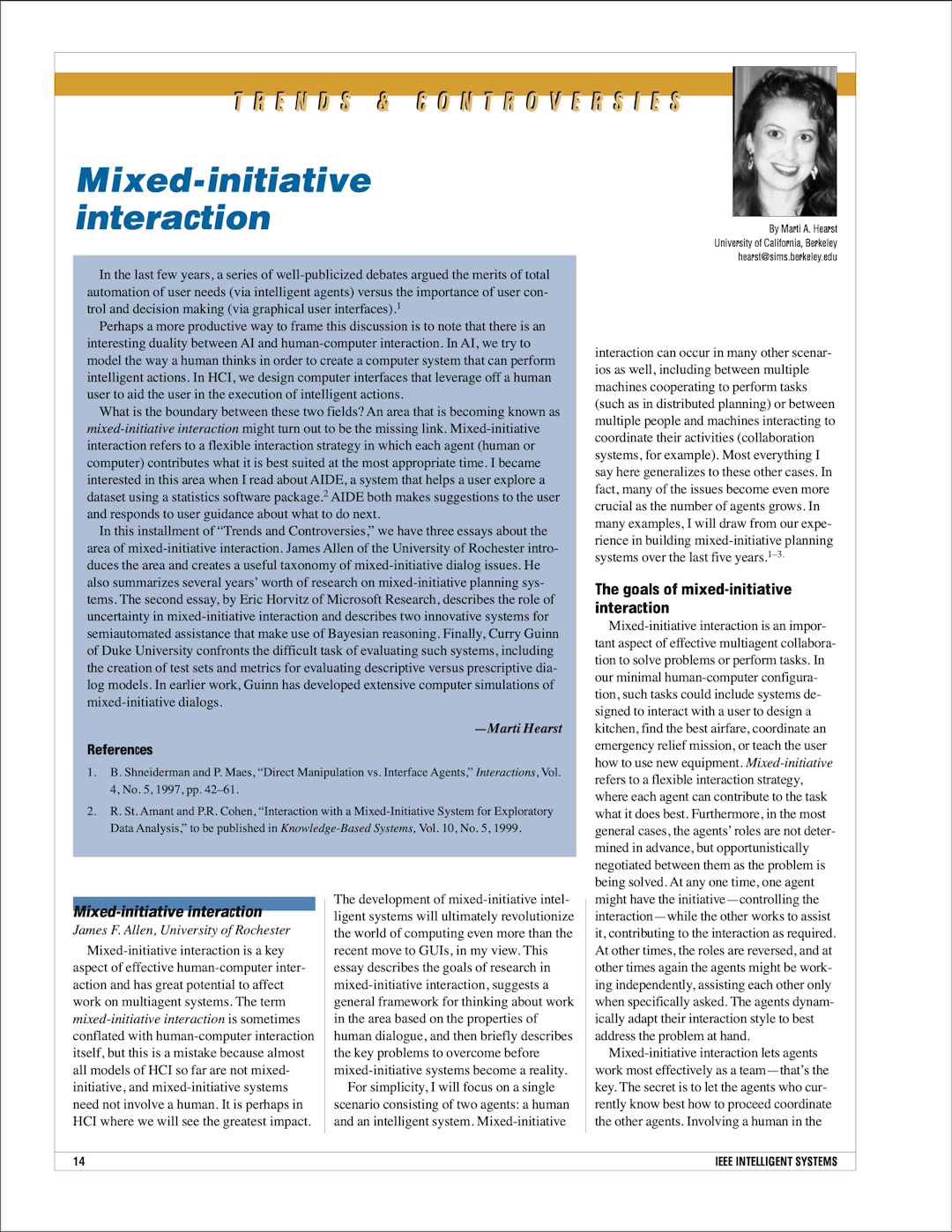
Mixed-Initiative Interaction
James Allen, Curry Guinn, Eric Horvitz, Marti Hearst · 01/10/1999
Published in 1999, the paper "Mixed-Initiative Interaction" by James Allen and colleagues serves as a landmark in Human-Computer Interaction (HCI) by introducing and elaborating on the concept of mixed-initiative systems. The paper argues that both humans and computers should be able to initiate actions and guide problem-solving in interactive systems, which stands as a departure from solely user-driven or system-driven models.
- Mixed-Initiative Systems: The paper presents the case for systems where both human users and computers can initiate actions. For HCI practitioners, this implies designing interfaces that enable two-way conversations, offering users more flexibility and control.
- Collaborative Problem-Solving: Emphasizing cooperative interaction, the authors discuss how mixed-initiative systems can assist in more effective problem-solving. This is particularly significant for complex tasks requiring nuanced human judgment and computational power.
- Adaptive Mechanisms: The paper touches upon the importance of adaptability in mixed-initiative systems. Systems should be able to learn from user interactions to offer increasingly relevant and targeted assistance over time.
- Context Sensitivity: Within mixed-initiative systems, understanding the context of user interactions is essential. The paper suggests employing robust context-recognition algorithms to facilitate more natural conversations.
Impact and Limitations: The paper’s ideas have influenced a variety of applications, from intelligent personal assistants to collaborative software in medical diagnostics. However, a limitation is the potential for "overstepping" by the system, where it might initiate actions that the user finds intrusive or unwarranted. Future work could focus on refining these adaptive algorithms to better align with user expectations and ethical considerations.
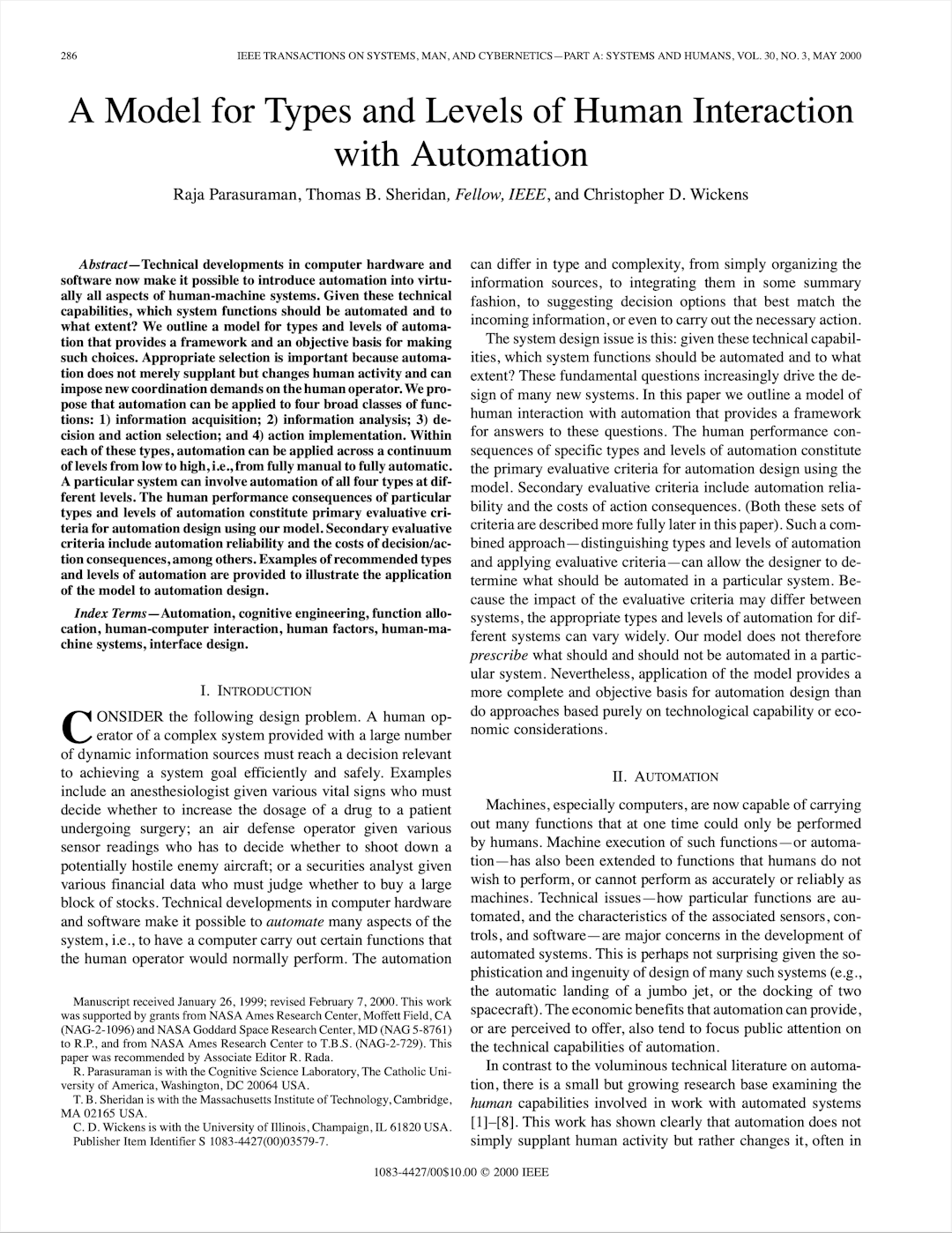
A Model for Types and Levels of Human Interaction with Automation
Raja Parasuraman, Thomas B. Sheridan, Christopher D. Wickens · 01/05/2000
The paper investigates human interaction with automation. It introduces a novel model for types and levels of human-automation interactions that has significantly impacted the field of Human-Computer Interaction (HCI).
- Types of Automation: The model identifies four types of automation: Information Acquisition, Information Analysis, Decision and Action Selection, and Action Implementation. Each type varies in its application and level of interaction.
- Levels of Automation: The authors introduce ten levels of automation; each differing in the amount of human operator involvement and decision-making. High automation levels result in decreased human engagement.
- Performance Effects: The study reveals that high levels of automation can degrade human performance resulting in human operators becoming complacent and out-of-the-loop.
- Automation Paradox: The authors uncover the automation paradox, where automation can solve complex tasks but introduce new errors and complexities.
Impact and Limitations: This model has stimulated significant research in HCI, exploring how interaction design can balance automation benefits while mitigating the drawbacks. Despite its contributions, the model does not account for changing user cognition and system dynamics over time, which necessitates further research.
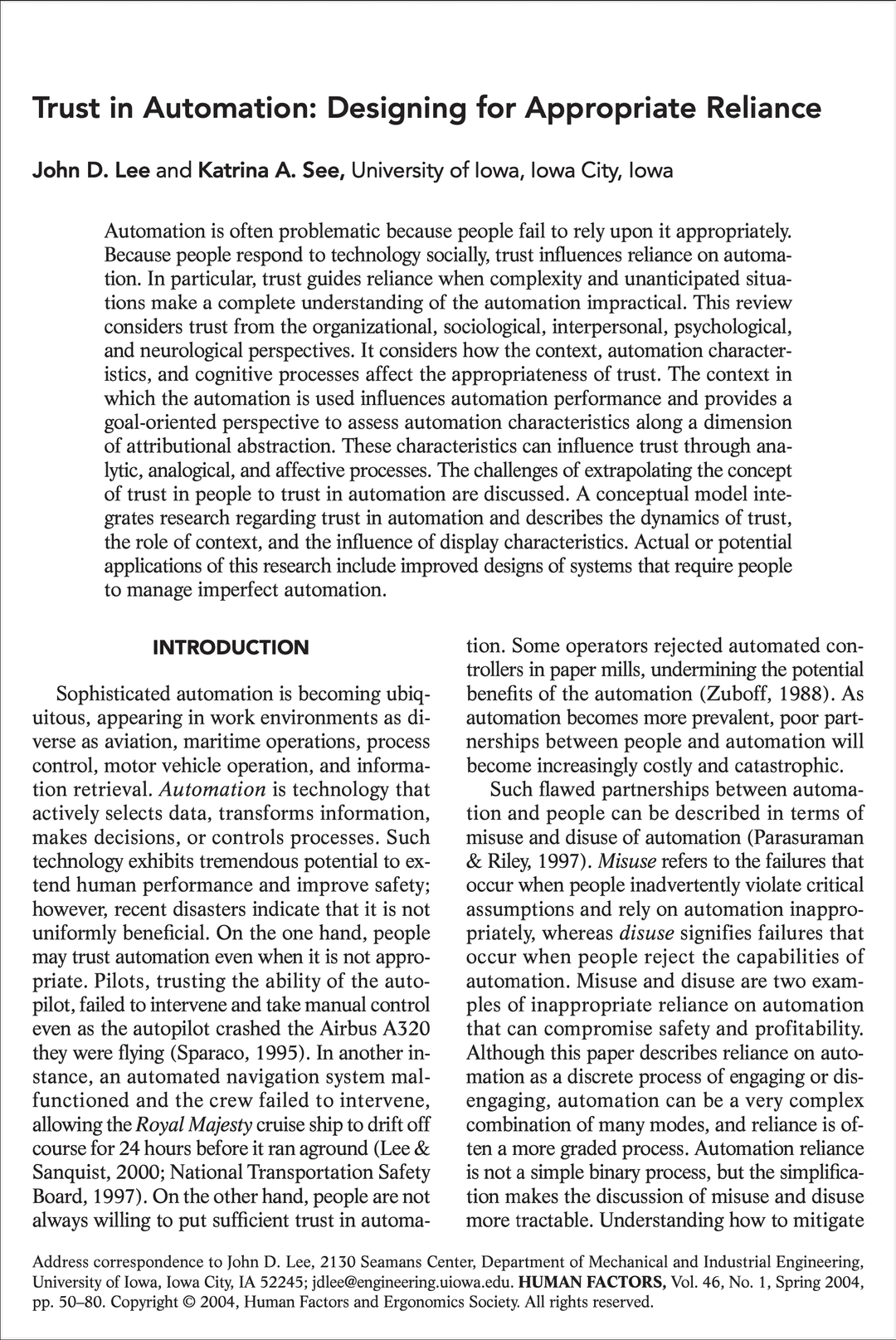
Trust in Automation: Designing for Appropriate Reliance
John D. Lee, Katrina A. See · 01/04/2004
John D. Lee and Katrina A. See's paper from the University of Iowa is pivotal in the discussion of Human-Automation Interaction (HAI). It explores how trust influences human reliance on automation, and offers a framework for designing systems that encourage appropriate trust levels, bridging the gap between psychology and engineering.
- Calibrated Trust: The authors emphasize the importance of calibrated trust, where the level of trust a user places in a system matches its actual reliability. For practitioners, this means designing feedback mechanisms that accurately represent system performance.
- Misuse and Disuse: The paper categorizes inappropriate reliance into misuse (over-reliance) and disuse (under-reliance). The aim is to balance system design to avoid both extremes, ensuring that automation complements human abilities.
- Dynamic Trust Model: Lee and See propose a dynamic model of trust that changes over time based on system performance and contextual factors. This is crucial for systems requiring long-term user engagement.
- Operator Training: The paper also discusses the role of training in cultivating appropriate trust. Effective training modules can help users understand system limitations and capabilities, leading to better decision-making.
Impact and Limitations: This work has had broad implications across various domains, including healthcare, aviation, and autonomous vehicles. However, the paper does not delve deeply into the ethical implications of manipulating user trust, a significant area for further exploration.
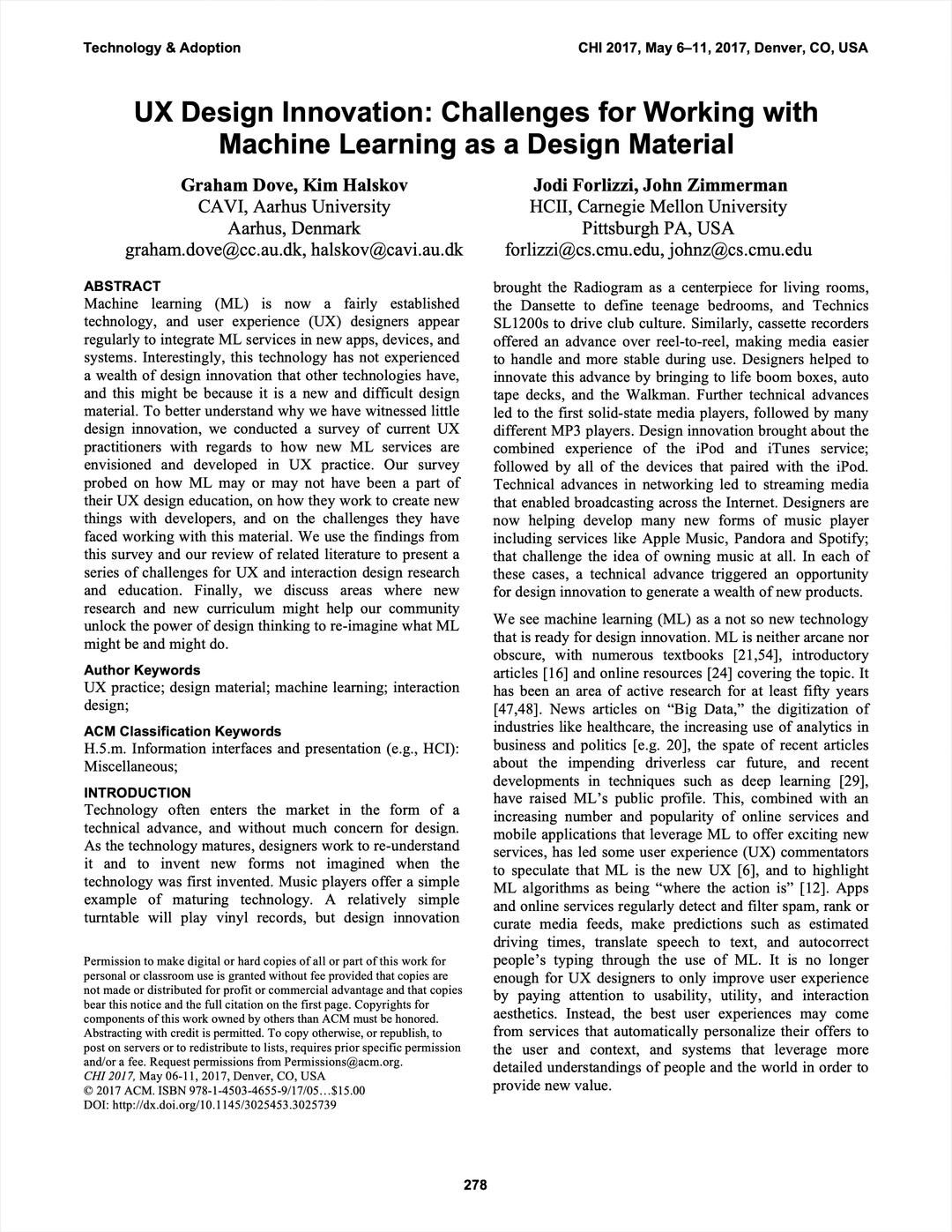
UX Design Innovation: Challenges for Working with Machine Learning as a Design Material
Graham Dove, Kim Halskov, Jodi Forlizzi, John Zimmerman · 01/05/2017
The paper focuses on the unique challenges that User Experience (UX) designers face when incorporating Machine Learning (ML) as a design material. It provides seminal insights into the crucial intersection of ML and HCI design.
- Machine Learning as a Material: The paper argues that ML should be treated as a design material, much like color, shape, or typography in graphic design. This view is critical as it shifts how designers approach ML in HCI.
- Design Challenges: Using ML as a design material introduces several distinct challenges. These include unpredictable outputs, the opacity of ML systems, and the difficulty of designing with data.
- Case Study Approach: Authors used real-world case studies to investigate these challenges. The practical examples offer clear implications for HCI designers navigating ML.
- Design Recommendations: The authors provide practical recommendations like prototyping data, fostering multidisciplinary cooperation, and adopting a more experimental mindset.
Impact and Limitations: The paper offers groundbreaking perspectives on approaching ML in HCI design, with immediate applicability for designers. However, the authors could further explore how the issues uncovered relate to other areas of AI, and how domain-specific challenges may alter the applicability of their recommendations. Future work could also discuss how to best educate designers on ML concepts.
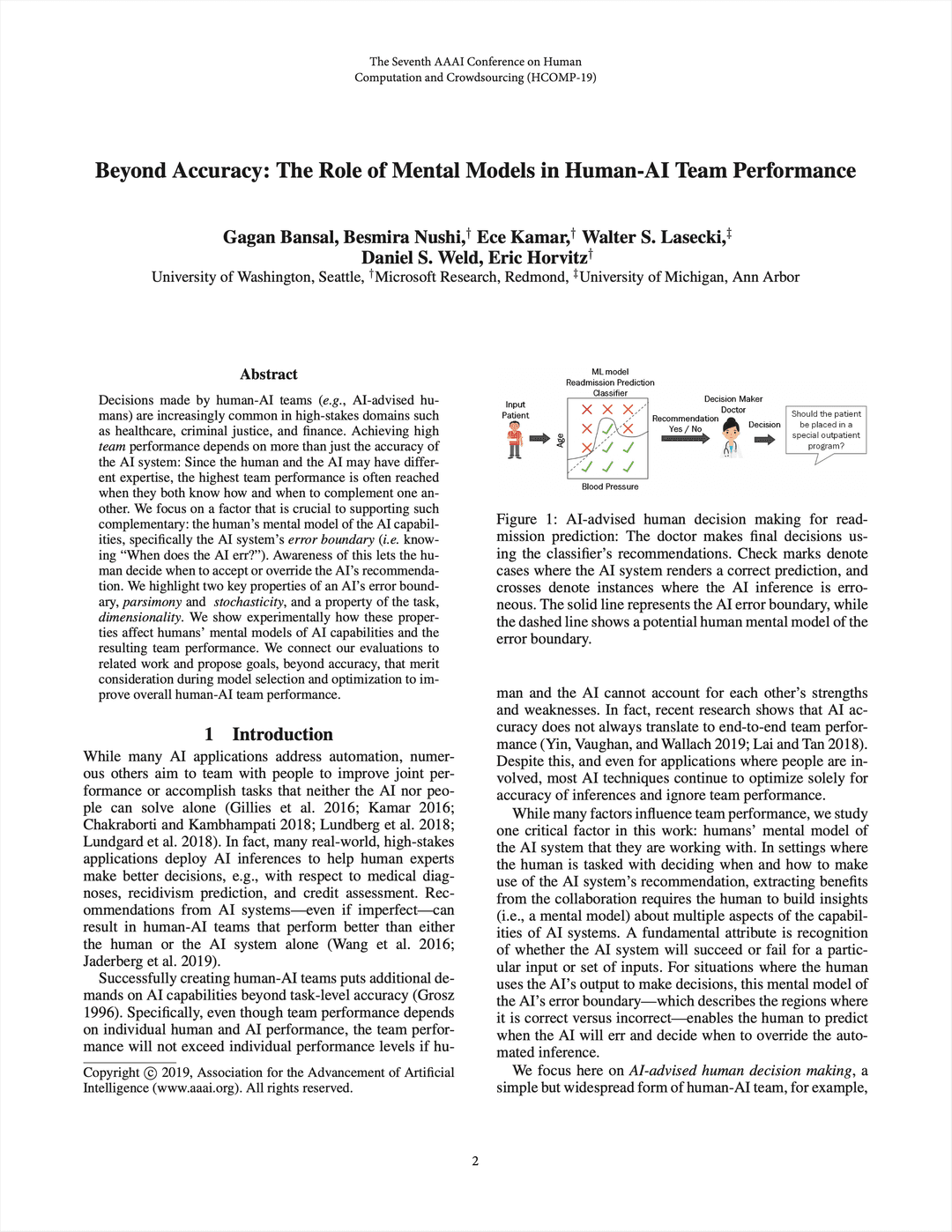
Beyond Accuracy: The Role of Mental Models in Human-AI Team Performance
Gagan Bansal, Besmira Nushi, Ece Kamar, Walter S. Lasecki, Daniel S. Weld, Eric Horvitz · 01/10/2019
This paper delves into the dynamics of Human-AI teams and the significance of humans' mental modeling of AI systems in building successful cooperation. The work delves deep into Human-Computer Interaction (HCI), pushing forward the understudied field of Mental Models in AI.
- Mental Models: The study proposes that understanding an AI’s limitations and capabilities, through dynamic mental models, can lead to improved human-AI collaboration.
- Communication Interfaces: The authors suggest that AI systems need to be designed with comprehensive communication interfaces that reveal enough system information to aid the development of accurate mental models.
- Training Vs. Showing System Uncertaintities: The research advocates for a balance between training users to predict AI responses and demonstrating system uncertainties to users.
- Empirical Evaluation: They conduct an empirical evaluation to show that when users understand an AI system's limitations, they work more effectively with the AI despite the system's accuracy level.
Impact and Limitations: This paper prompts HCI researchers and AI practitioners to focus more on facilitating the human understanding of AI. By ensuring humans can predict AI behaviour and understand system uncertainties, they can foster better collaboration. However, it doesn't deeply explore how different types of AI (ML-based, rule-based, etc.) might require different approaches for developing mental models. Further research is suggested in this area.
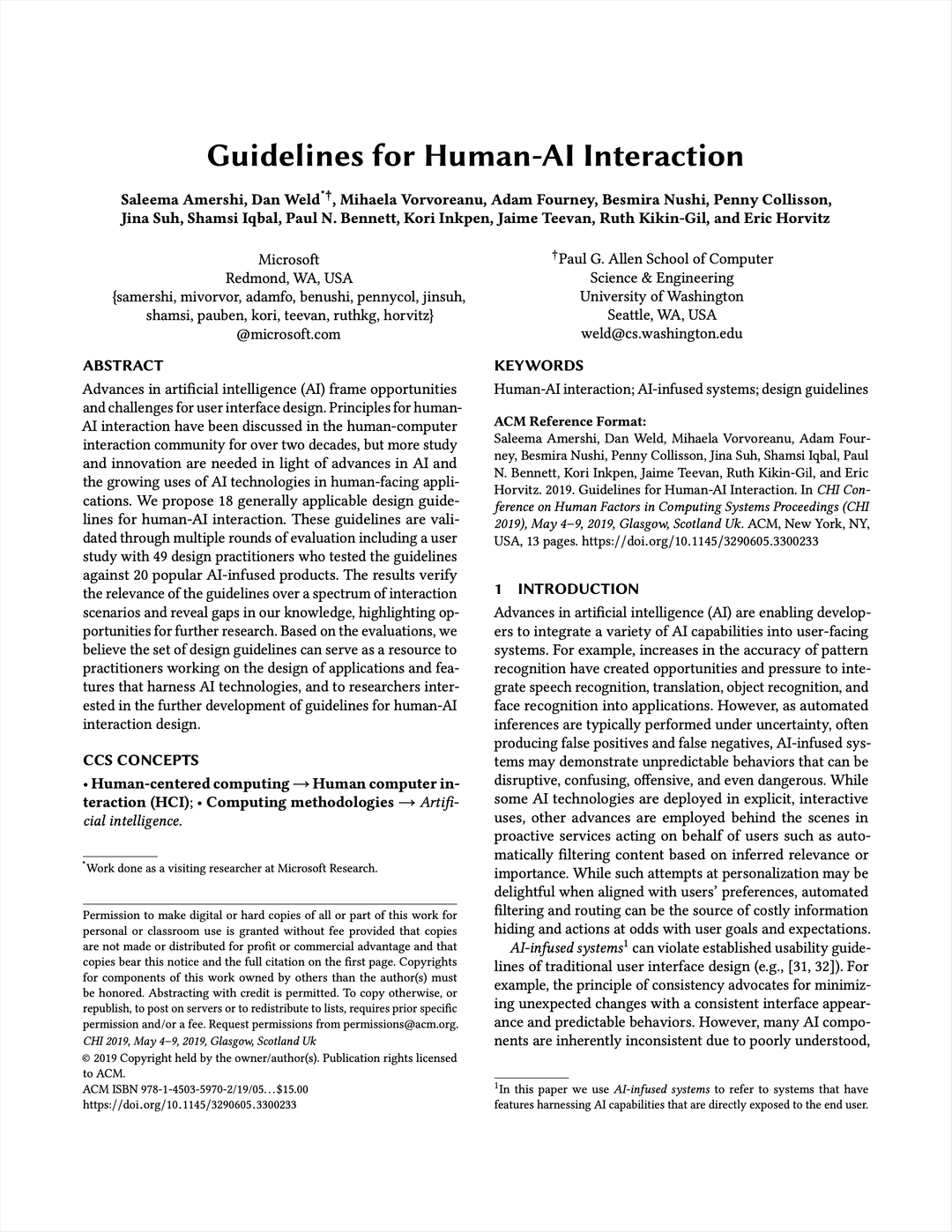
Guidelines for Human-AI Interaction
Saleema Amershi, Dan Weld, Mihaela Vorvoreanu, Adam Fourney, Besmira Nushi, Penny Collisson, Jina Suh, Shamsi Iqbal, Paul N. Bennett, Kori Inkpen, Jaime Teevan, Ruth Kikin-Gil, Eric Horvitz · 01/05/2019
The paper spotlights the necessity for actionable guidelines in Human-AI Interaction (HAII), offering a vetted list of 18 guidelines developed through a consensus of a wide cohort of Microsoft's researchers and engineers.
- User Perception and Expectation: The paper posits that the usability of AI systems significantly depends on meeting user expectations and understandings of system functionality.
- Transparency and Control: Emphasizes the importance of clarity in AI systems' behaviors and providing the user with ample control over interactions, fostering trust.
- Guided Learning and Error Handling: AI systems should guide users to enhance their models and show appropriate responses if they learn incorrectly or can't complete tasks.
- Keeping User's Goal Primary: AI systems should always keep the user's main task cardinal and ensure minimal distractions while providing assistance.
Impact and Limitations: The guidelines, while geared towards Microsoft, have far reach applicability across the backdrop of emerging AI technologies, streamlining human-computer interactions. Limitations persist in the form of abstract guidelines, requiring specific contextualization in practical applications. Future work could include refining these guiding principles with empirical validation in diverse AI contexts.
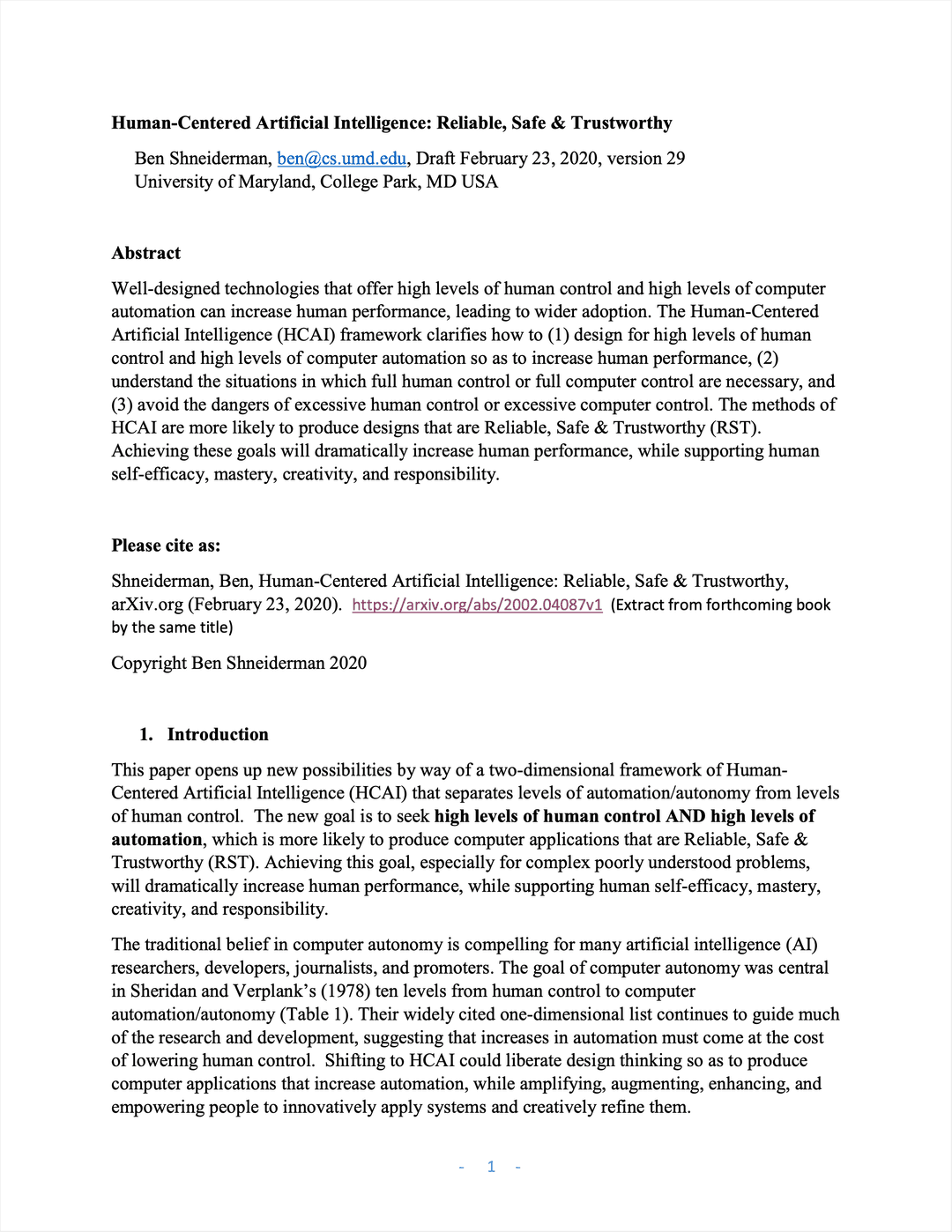
Human-Centered Artificial Intelligence: Reliable, Safe & Trustworthy
Ben Shneiderman · 01/02/2020
The HCI paper centers around the creation and control of Artificial Intelligence (AI) from a human-computer interaction perspective. It proposes a human-centered approach towards AI to increase its reliability, safety, and trustworthiness.
- Human-Centered AI: The paper advocates for machine learning methods in HCI, promoting trust, and initiating the need for improved user interfaces for AI developers and users.
- Reliability and Safety: Shneiderman emphasizes the necessity for AI to be reliable and safe, recommending stricter testing and debugging processes.
- Trustworthy AI: A focus on stringent controls, the production of predictable results, and appropriate responses in the event of failure is proposed to instill greater trust in AI systems.
- Improved User Interfaces: Constructive suggestions are presented to enhance user interfaces for both programmers creating AI and those utilizing AI, providing a more comprehensible experience.
Impact and Limitations: The paper generates broad implications for HCI and AI fields. By weaving ethics into AI, we can advocate for a world where AI is trusted, safe, and beneficial. The paper, however, doesn't provide practical ways of achieving its recommendations. This calls for further research and exploration on how AI can become more human-centric while maintaining its mathematical prowess.
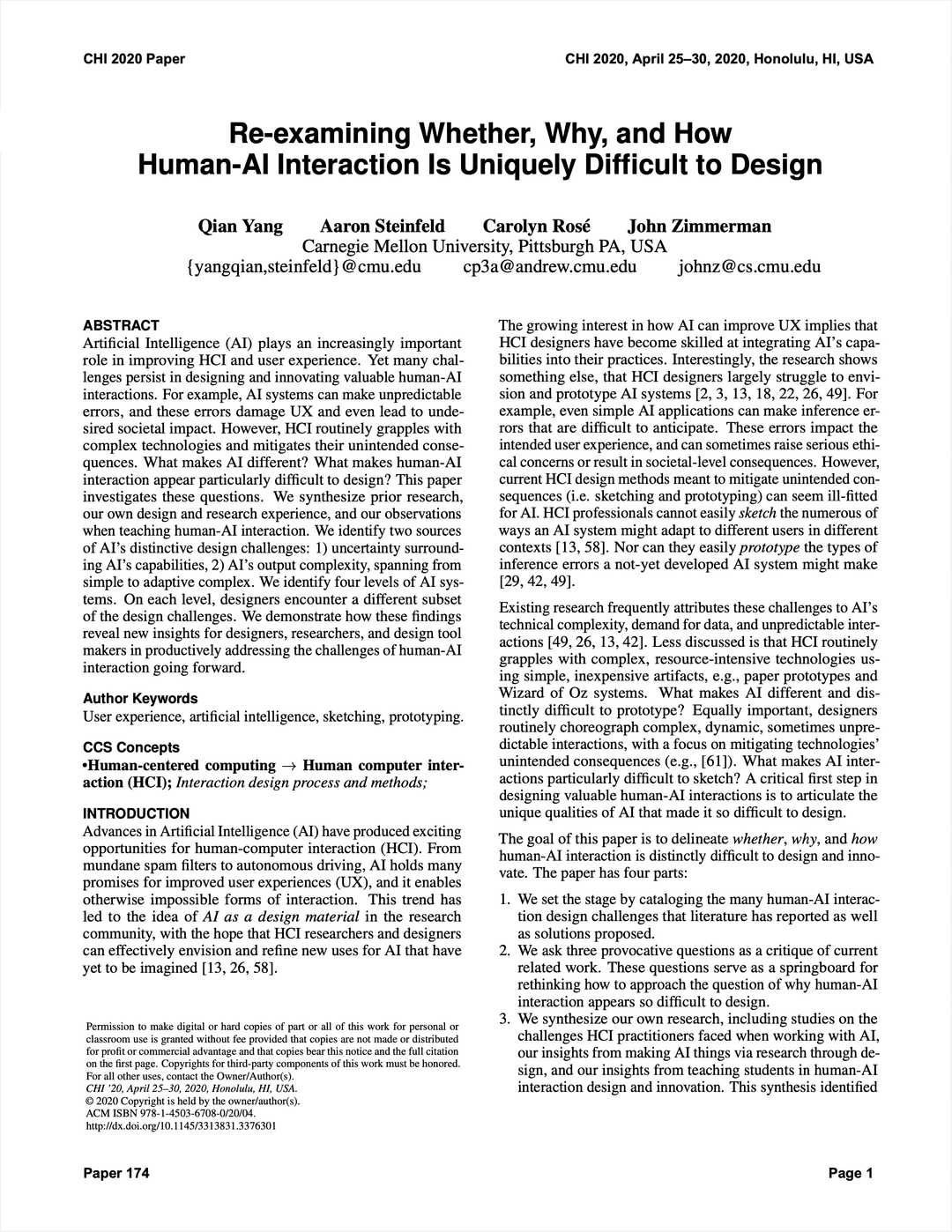
Re-examining Whether, Why, and How Human-AI Interaction Is Uniquely Difficult to Design
Qian Yang, Aaron Steinfeld, C. Rosé, J. Zimmerman · 01/04/2020
This seminal HCI paper investigates the distinctive challenges posed by Human-Artificial Intelligence (AI) interaction design. The authors push the boundaries of HCI research by questioning prevalent normalized concepts in AI interaction strategies.
- Disparity in Design and Effectiveness: AI designs often struggle to deliver the predicted benefit due to the variances in user reactions and expectations.
- Dependency on Data: Rather than static design, AI interaction design adapts to the data it encounters. This evolving nature creates unpredictability and inconsistency.
- Accountability Absence: Difficult to incorporate human-like accountability in AI-systems as these systems lack explicit cause-effect logics.
- Ethical Dilemmas: The risk of privacy invasion, prejudice reinforcement, and potential misconduct amplifies the intricacies of AI design.
Impact and Limitations: Advances in Human and AI interactions have far-reaching implications for HCI, yet these practices aren't devoid of complexity. This paper's critical takeaways enlighten designers and practitioners about inherent difficulties in AI design, leading to more anticipatory and reflective design practices. Future work can further explore ways to harmonize human values with evolving AI mechanisms.